P value: What's Chance Got to Do With It?
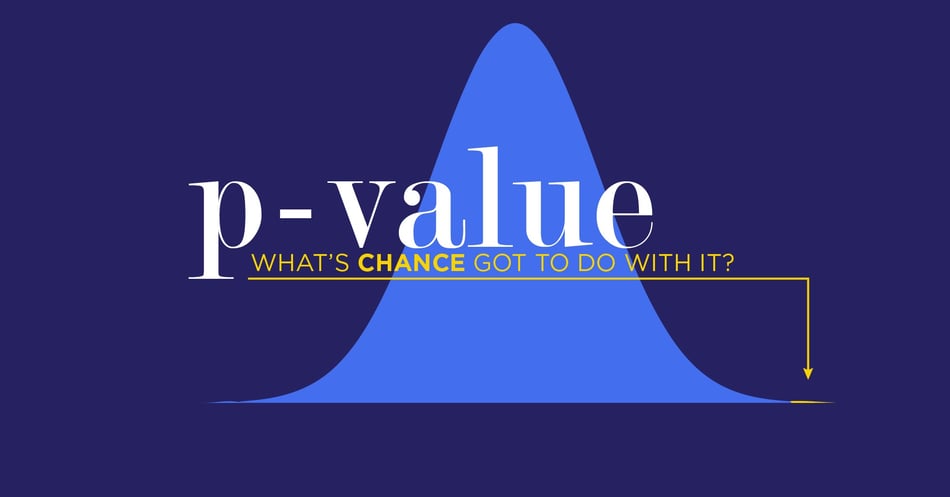
In scientific research, we test interventions to a problem and then measure the result: Did a medication improve patient outcomes? Did a training program lead to improved hand hygiene? Did copper-impregnated materials reduce the number of healthcare-associated infections? Simple before-and-after measurement is not enough when it comes to generating strong evidence. While the patients, the hand hygiene, and the infection rates may improve, it is vital to demonstrate that the intervention being studied was the cause of that improvement. How does a researcher demonstrate strong evidence? This post will explore the statistical representation of strength of evidence: The p value.
In any given study, a researcher is testing the impact of a variable on a result. For example, a researcher wants to see the impact of copper-impregnated materials on HAI rates at a hospital. HAI rates are collected from the hospital before installation of the copper-impregnated materials, and then compared with rates after the materials have been installed. These data points are run through many statistical analysis tests to determine whether there is a difference in the rates and if that difference is significant (big enough to mean there is a connection between the variable and the result). A significant reduction in HAI rates would mean that the materials, the tested variable, played a role in the reduction.
The P Value
A p value, or probability value, is provided alongside the results to show how likely it would be to observe those results if left completely to chance. The p value is represented by a number less than 1, with 0 meaning “0 likelihood that you’d see these results if left to chance” and 1 meaning “100% likelihood you’d see these results if left to chance.” The lower the p value, the greater likelihood that the tested variable did have an impact.
Let’s take our example of the copper-impregnated materials and HAI rates. If there is a reduction in HAI rates, and the statistical tests show that there is enough of a reduction to show that the variable, the materials, had an impact, then you would want to know what the chances are that you’d see that same reduction without the materials, if they’d never been installed and the hospital just went on as it had before. The p value would tell you that – the lower the number, the smaller the chance that the reduction was due to chance alone.
Effect Size
One thing to keep in mind is the number of data points in a study, because this does impact p value. The more data points you have (more observations, more participants, more measurements), the easier it is to prove that any impact – even a small impact – was not due to chance. If you have fewer data points – a smaller sample size – you need a huge difference in order to conclude that your observed results are not due to chance. Let’s look at how this plays out.
If your sample size is | And your difference in outcomes is | Then your p value (probability the results are due to chance) is | And your strength of evidence is |
Small | Huge | Low | Strong |
Small | Small | High | Weak |
Large | Huge | Very Low | Very Strong |
Large | Small | Low | Strong |
The advantage of having a large sample size is that you reduce the probability that your results are due to chance alone.
The Null Hypothesis
“What are the chances that you’d have these results if the variable had had no impact?” Why use such a convoluted way of thinking? This has to do with the idea of the null hypothesis, the hypothesis stating that the variable has no impact on the results. The p value is defined as “the likelihood of getting the observed results if the null hypothesis were true.” In our example, the null hypothesis is “Copper impregnated materials have no impact on HAI rates.” The p value, therefore, shows the likelihood that we’d get reductions in HAIs if the null hypothesis were true, that is, if the materials had had no impact. You’d want a low chance of this, right? So that’s why a low p value is a good thing.
A Simple Conversion in 2 Steps
So this brings us to a simple conversion anyone can do in their head when confronted with a p value: 1) Convert it to a percentage and that is the % probability that you’d see those results due to chance alone. 2) Remember the number .05: This is the cut off point for strong evidence accepted by scientific publications and the scientific community as a whole (although there is some discussion about using p values at all). This cut off point means that anything less than .05, or a 5% probability that the results are due to chance alone, is considered strong evidence.
P value |
% probability that results are due to chance alone |
Strength of evidence |
Less than 0.01 | Less than 1% | Very strong |
0.01 to 0.05 | 1% to 5% | Strong |
0.05 to 0.10 | 5% to 10% | Some weak evidence* |
More than 0.10 | More than 10% | Little or no evidence* |
* A bigger sample size could show stronger evidence.
Statistical analysis is an enormous topic full of nuance, variation, context, and methodology. Happily, you don’t have to understand what happens behind the scenes in order to make your own educated analysis of the data you read in a scientific journal or marketing publication. We hope this introduction to p value has helped you become a more confident consumer!
Editor's Note: This post was originally published in September 2016 and has been updated for freshness, accuracy and comprehensiveness.