How are Graph Neural Networks Being Used in the Fight Against Healthcare Acquired Infections?

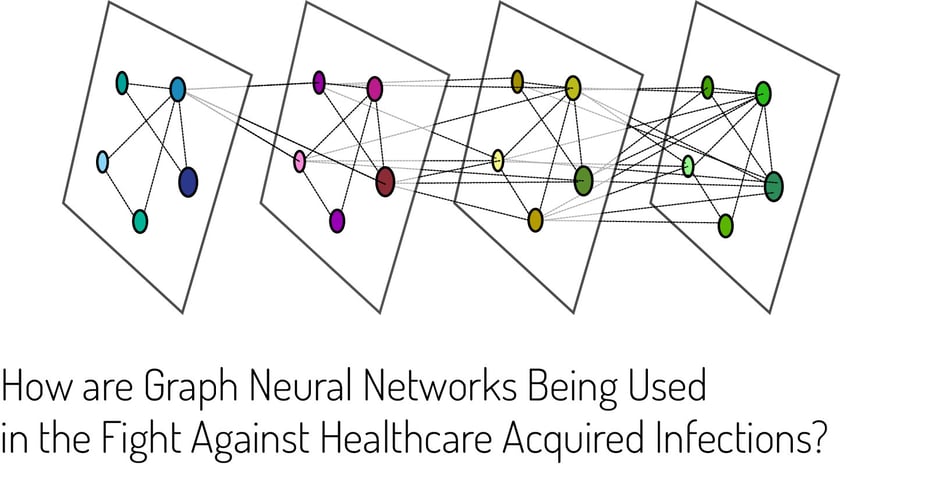
Graph Neural Networks (GNNs) are computer algorithms that take data from graphs and uses relationships between the data points to make predictions or recommendations. Much like our own brains, neural networks connect and correlate information to generate possible patterns that can be helpful to a variety of fields, including infection control and prevention (IP). In IP, GNNs are being used to predict risk for healthcare acquired infections (HAIs) and make decisions about resource allocation. In today's post, we'll take a closer look at how this is being done.
A graphic neural network is a way to use machine learning to replicate how our own brains work to use data to reach conclusions. In our own organic neural network (our brain), we compare and contrast data across a wide variety of sources, taking bits of what we learned in one context and applying them to what we are learning in a new context. The GNN attempts to use computers to do the same thing. Data points, called nodes, are fed into the algorithm, along with relationships, or edges between data points. The resulting network structure represents all the nodes and edges and incorporates layers, that is, additional networks that can then be related to each other. The result of all these interconnections is a readout function, also called a pooling function, which are the predictions or decisions based on the GNN as a whole.
How can GNNs be used in IP? Data collection pervades every aspect of the field of infection control and prevention. Hospitals collect data on patient, community, and staff demographics. Medical records store data on diagnosis, prescriptions, treatments, medical caregivers, locations, vital statistics, and outcomes. IP collects data on hand hygiene compliance, use of PPE, staff training, device usage, and infection rates. A GNN comes in and aggregates all that data into one complexly interconnected network that can find patterns unseen by the eyes of mere mortals. Here are a few examples:
- GNN using patient demographics across a wide variety of clinical features predicts risk of HAIs, resulting in better treatment plans for individual patients. Link here.
- GNN using social network structure and data about disease transmission reconstructs spread of epidemic with 70% accuracy, which can inform future predictions. Link here.
- GNN assess risks of patient colonization by multi-drug resistant Enterobacteriaceae by part of body, patient/staff interactions, and other spatiotemporal features. Link here.
Machine-based learning and the resulting predictive possibilities will become more and more sophisticated and accurate as these modeling systems improve and become more user-friendly. As we become more adept and leveraging the abilities offered by these neural networks, we will become more efficient at predicting and addressing risks of acquiring a healthcare associated infection. Is your facility beginning to use machine learning or other predictive software in your fight against HAIs? Tell us about it in the comments below!